As a company we are always focussed on the performance of our core web sites. However, with Google recently announcing that Core Web Vitals will be used as signals to the SEO indexing on mobile, the performance became the number one priority. Having figured out that React Client side App may not be the best technology to achieve the Core Web Vitals in our case, we decided to jump on to the Server Side Rendering bandwagon. This blog is about how we achieved the migration of our React Client side app to Nextjs based Server Side Rendered App. Our partner Epsilon Delta helped and guided us to achieve the below performance parameters.

Measurement Standards and Tools –
We used two kinds of measurement during the duration of our engagement.
Webpagetest by Catchpoint – For Synthetic measurement, we used the Webpagetest, as it provides a paid API interface to measure performance of pages synthetically from a specific browser, location and connection. You can store the data from each of the runs in your db and build a frontend to see the reports. While the advantage of webpagetest is that it provides a visually nice screen with all relevant performance parameters and waterfall for each run, it can not replace what Google is going to see for real users for all variations like network, browser, location etc. It is also difficult to capture performance experience of the logged in users through webpagetest as it requires a lot of scripting.
Gemini by EpsilonDelta – For real user measurement, we could not rely on just the Search Console or the Lighthouse or PageSpeed Insights, as primarily the real user data (the field data) is fetched from the Chrome User Experience db (Crux DB). The result set is generated based on the 75th percentile of the past 28 days data. Thus, instantaneous performance results are not available in Crux db once you push the performance optimization to production. It will be between 28 days to 56 days to know if the performance change helped in achieving the goal or not. In order to get the real time, real user Core Web Vitals, we decided to use the Gemini Rum Tool by Epsilon Delta.
Another advantage of Gemini is that it provides the data aggregated on page templates, url patterns and platform automatically. So we were able to identify the top page templates which need to be fixed on priority.
Key Performance Issues Encountered
Before November 2020 , Google was focusing on First Contentful Paint (FCP) as the most important parameters for the performance. However this changed when they announced the Core Web Vitals Concept, i.e. First Input Delay (FID), Largest Contentful Paint (LCP) and Cumulative Layout Shift (CLS).
The FID is approximately representing the Interactivity of the web App.
The LCP is approximately representing the Paint Sequence of the Web App.
And the CLS is representing the page layout shifts or movements within the visible area of the page.
While the our site had the FCP in the green zone, the LCP and CLS were the newer performance parameters. The LCP for the our site was around 5.3 seconds and CLS was 0.14 for the mobile experience.
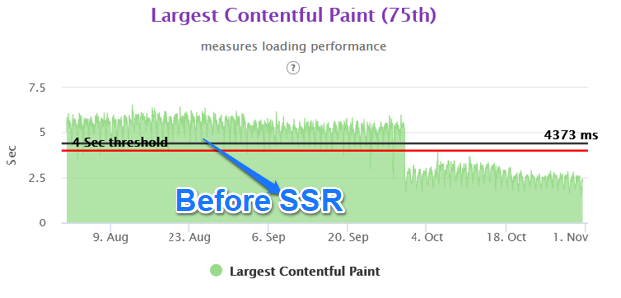

The then React Client side App codebase was having many inefficiencies, which resulted in having a large number of js and css files. The overall resource request count on each page was in excess of 350 requests. There were many other issues with the React Client side app code, where the configuration and the structure was not out of the box. There were a large number of customizations applied on the code, which made the code base very complex. Fixing the existing issues and then having desired performance level did not seem prudent and we decided to try the latest technology of Server Side Rendering.
Why SSR (Server Side Rendering) ?
The detailed analysis of the then performance numbers provided us with the following observations.
Unplanned Layout Shifts – The Layout Shifts were happening as the Client side javascript was adding or removing the page structures as per the data coming from APIs and in order to not have the layout shifts, we would have needed to add a lot of placeholders. However in order to add the placeholders and attributes in the html, we needed to have the above the fold html of the structure served from the server side in the basic html first. This is not the case for most of the Client Side served architecture.
Delayed LCP – The Largest Contentful Paint was degraded as the LCP image was not loading earlier in the waterfall. However, even if we would have preloaded the LCP image, the base container for the LCP image was not present in the html. Hence the LCP performance would never have reached our desired level. Thus, in order to solve this problem, we needed to have the above the fold html served from the server side. Hence we started looking at the Server Side Rendering technologies.
Why NextJS ?
During this time, NextJS was already popular and some of the other sites had already tried building the web app using NextJS technology. There were quite a number of articles available to assist in building the site on Nextjs. Following features were very useful to decide the move to NextJS.

Launch of NextJS 11
The biggest reason we moved to NextJS is the release of Nextjs version 11.This version provided the ability to handle CLS of the server side rendered code. There was a demo and migration system available to migrate the React Client side app code to Nextjs Server side app code. Of Course that migration works only with certain conditions, but luckily for us, our React client side code fulfilled all the requirements.
The version has improved performance as compared to version 10. It has features like Script Optimization, Image Improvements to reduce CLS, image Placeholders to name a few. More details are available at [2].
https://nextjs.org/blog/next-11

Migration Planning and considerations
In order to do a neat migration, you need to consider the following tasks before you begin the migration.
Page Level Migration –
Nextjs handles the pages on url patterns only. So we decided to move our top 3 high trafficked templates Product Details Page, Product Listing Page and Homepage sequentially. Each of these pages were being served through a url pattern.
Service Worker –
In any domain, there can be only one service worker. Since our React Client side app already had a service worker, we needed to ensure that the same service worker was copied to NextJS code on Production deployment. We planned static resource caching based on the traffic share being served from the 2 code bases. I.e. React Client side and Nextjs server side. So initially the service worker was served from React Client side code. Once 2 pages were migrated to Server Side, we moved the service worker to Nextjs code base.
Load Balancer –
On Load Balancer as well, one needs to ensure that proper routing happens for url patterns which are getting migrated. Fortunately modern load balancers provide plenty of options to handle the cases.
Soft to Hard Routing –
The basis of the PWA app was to provide a soft routed based experience for pages after the landing pages for the users. However, with 2 code bases, the internal routing would have caused the issue. One needs to disable the page level soft routing for the page which is being developed for moving to a new code base. This way you can ensure that the request is always reaching to the load balancer for effective routing. Once the migration is complete for each page, you can move the routing back to soft.
SEO Meta data –
As in any new code development, one needs to ensure that all the SEO meta data is present in the page as compared to the existing page and a quick check can be done by running Google SEO url check.
Custom Server –
A custom Next.js server allows you to handle specific URLs and bot traffic. We wanted to redirect some urls to a new path & redirect bots traffic to our prerender system. Next js has a redirects function which can be configured in next.config.json by adding a JSON object. But as our list of urls is dynamically updated via database /cache, we added logic in the custom server. In future with Nextjs12 we will move this logic to middleware.
API changes –
During the migration we ensured that the existing APIs will be used to the maximum extent in the new Nextjs code. Fortunately our APIs were already designed in a way that the output was separated out for above the fold and below the fold data. Only in cases where we wanted to limit the data coming through the APIs and any configuration was not possible, that we created new APIs.
JS Migration –
We started with creating a routing structure using folders and file names inside pages directory. For example, for the product details page, we used dynamic routes to catch all paths by adding three dots (…) inside the brackets.
We copied our React components JS files related to a particular page from the existing codebase to the src folder in the NextJS codebase. We identified browser level functions calls and made changes to make them compatible with SSR.
Further, we divided our components by code usage between above the fold & below the fold area. Using the dynamic importing SSR false option, we lazy-loaded below the fold components. Common HTML code & some third party library code was added in _document.js
CSS Migration –
We imported all common css files used on our site like header.css, footer.css & third party css inside pages/_app.js file. Component-level css used for styles are used in a particular component. Components.module.css files are imported inside the component js file.
Performance Optimization Considerations
Performance is a very wide term which is being used in the technical community. The term is used in various aspects like backend performance, database performance, CPU, memory performance, javascript performance etc. Given that Google is heavily investing and promoting the concept of Core Web Vitals, we decided that the focus of our performance goal will be core web vitals.
We also firmly believe that even though FCP is not the Core Web Vitals anymore, FCP is equally important for perceived user experience. Post the url entered on the browser, the longer the blank white screen shows to the user, the more is the chances of the user bouncing. While we had already achieved a certain number for our FCP performance, we wanted to ensure that the FCP does not degrade much on account of Server Side Rendering
Server Side vs Client Side –
Thus, it was important for the html size to be restricted, so that the html generation time on the server is less and FCP is maintained. We painstakingly moved through each major page template and identified which page components are fit for Server Side Rendering and which are fit for Client Side rendering. This helped in reducing the html size considerably.
Preconnect calls –
Preconnect is the directive available which directs browsers to initiate DNS connect and SSL in case of domains. This helps in saving the initial time before the actual resource fetch.
<link rel=”preconnect” href=”https://fonts.gstatic.com” crossorigin=””>
Preload LCP image –
LCP being the most important parameters in the core web vitals, it becomes very important to identify the LCP at the time of designing the page and identify if the LCP is caused because of an image or a text. In our case, it was the image which was causing the LCP. We ensured that the image is preloaded using the preload directive.
<link rel=”preload” as=”image” href=”https://img7.hkrtcdn.com/16668/bnr_1666786_o.jpg”>
Making CSS Asynchronous –
CSS is a render-blocking resource. We wanted to inline critical css used in above the fold area of the page & make all css tags async to improve FCP and eventually LCP.We used critters npm module which extracts, minifies, inlines above-the-fold CSS and makes CSS link tags async in server side rendered HTML. During the implementation, we found an issue with the plugin while using the assetPrefix config for CDN path. The issue was causing only base domain urls for static resources to be used in the plugin. There was no option for CDN urls or it was not working. While we raised the issue with the NextJS team, there was no fix available. So we added a patch in our code to include CDN urls for static resources. As of now, the issue has been fixed by the NextJS team and the fix is available in the latest NextJS stable version.
Reducing the Resource Count –
In the NextJS project, we moved from component based chunks to a methodology where the js and css files were split up based on global code where common functionality was combined for above the fold working and local code where non common functionality was combined. We also ensured that the above the fold javascript was rendered server side vs below the fold on client side. This helped reduce the resource count on the Product Listing Page from 333 to 249 and on the Product Details Page from 758 to 245.
Javascript size reduction in Nextjs –
We did a thorough analysis of the javascript used at the server side and client side in order to identify unused javascripts, analyze which components and libraries are part of a bundle, and check if a third party library showed up unexpectedly in a bundle. There are few possible areas of improvement, e.g. Encryption algorithms used in the javascript code if any. We ensured that no third party js is integrated in the server side js except when it’s absolutely necessary and added as inline code.
We used Next.js Webpack Bundle Analyzer to analyze the code bundles that are generated but appear to be unused. this tool provides both server side & client side reports in html file this helps to inspect what’s taking the most space in the bundles

Observations –
Post completion of the project, we can identify a few key points related to our goal of core web vitals improvement.
Pros
Performance Optimization –
We were able to achieve close to 50% improvement in real user performance for LCP and bring it very close to 2.5 sec. This was possible because we used the server side rendered technique to get the very element in html causing the LCP.
The Layout Shifts on the client side also reduced to a great extent as we were stitching the page on the server side for above the fold area. Thus any major movements in CLS were not happening in the client side area.
Greater control on served experience –
Because of the server side rendered experience, we were able to decide the experience which we wanted to serve to the user at the time of html generation. So we had better control on what elements we wanted to show and what not. Most importantly this also helped us decide on our category based views and type of user based view. .i.e admin, premium vs normal user etc. In the client side environment, this very problem caused a lot of complexity in our earlier React based code.
Cons
Higher backend latency –
One of the tradeoffs of moving to server side rendering is the higher backend latency. If not managed correctly, it can degrade your FCP and by that means also degrade the LCP. One needs to carefully consider the structure of the page to ensure minimal increase in the backend latency. We did this by moving the below the fold functionality to client side rendering technique. Thus html generation time was reduced. We also created or modified existing API giving precise json data for backend integration. Better API response time helped us in reducing the backend latency. We also reduced the state variable size, as in nextjs, the state variable comes in the html. Larger state variables will cause the html size to increase.
Lesser support from Tech Community –
When we started working on the project, NextJS was relatively newer technology and lesser documentation was available. There were limited technical issues that were mentioned in various forums or the community had not brainstormed on the possible solutions by that time. However, this is not the case anymore and NextJS is a widely accepted technology.
Infrastructure Investment –
One of the other areas which need attention is the sizing of the backend infrastructure and possibly the infrastructure on API side. By design, the server side rendering moves the processing to the servers. Thus naturally one needs more capacity servers to serve the front end code as compared to the React client side app. We believe this is a small price one needs to pay to improve the Core Web Vitals.
Summary
With the Nextjs migration, we were able to achieve the performance goal for Core Web Vitals in the fixed amount of time having no major slippages. The result graphs are as follows.
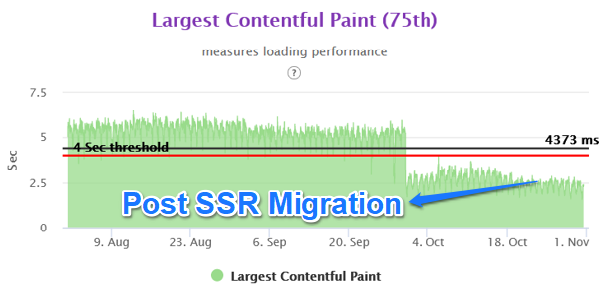
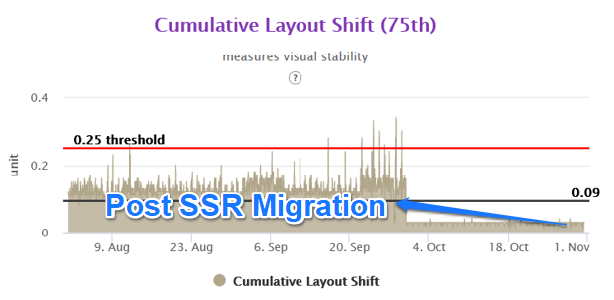
Mobile Homepage –

Mobile L1 Category Listing –

Mobile L2 Category Listing –
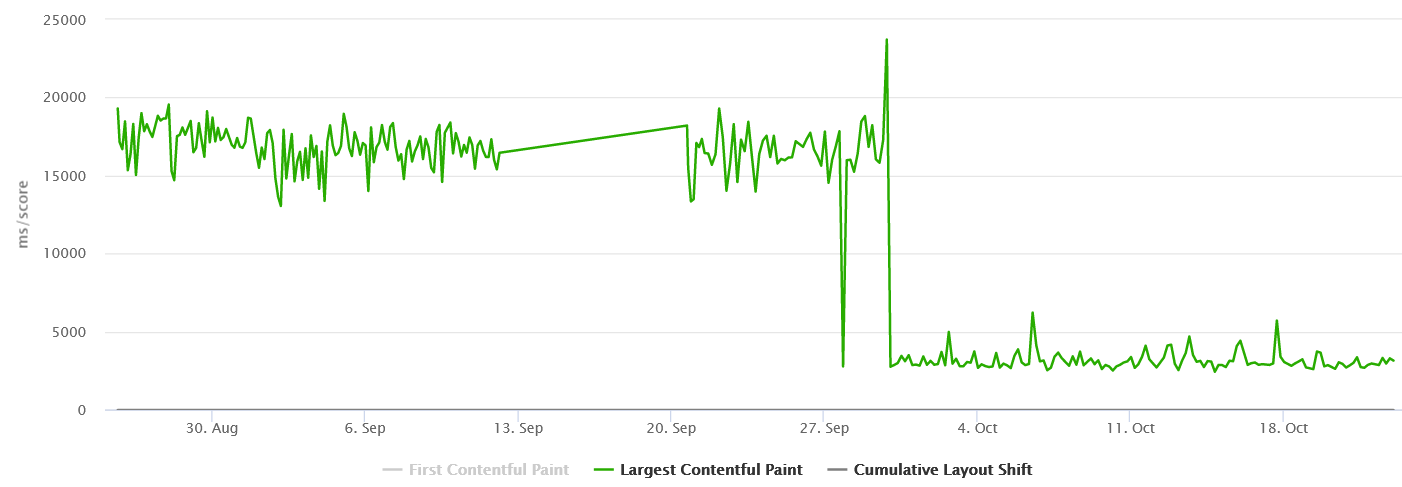
Mobile Product Details Page –

LCP 75th Overall in Gemini
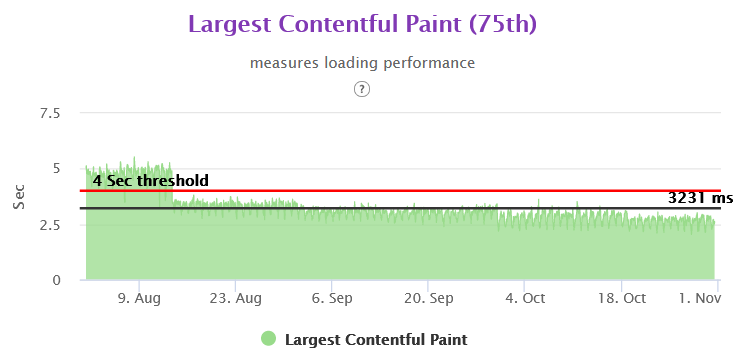
CLS 75th Overall in Gemini

LCP PDP Mobile 75th in Gemini

CLS PDP Mobile 75th in Gemini

LCP Category Listing Page 75th Gemini

CLS 75th Category Listing Page Gemini

Homepage 75th Gemini
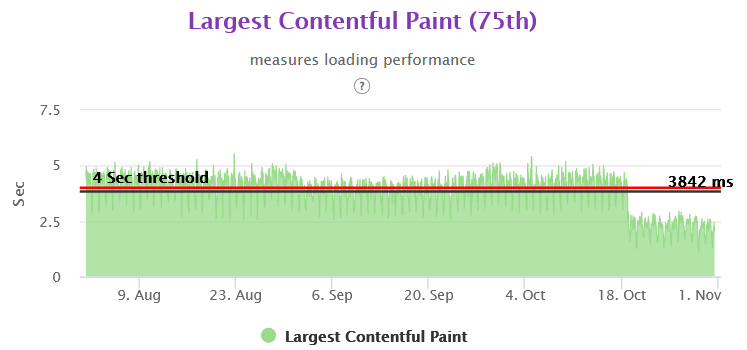
CLS Homepage 75th Gemini

The above content is an outcome of our experience while working with above problem statement. Please do feel free to reach out and comment in case of any feedback and suggestion.